Let’s assume that our data is stored on the Kafka cluster and it should be moved to another storage layer which is will be HBase in this case. And few transformations need to be made before data is moved. These steps can be depicted as below architecture.

Data could only be collected using the Spark streaming application without Kafka. But, Kafka as a long term log storage is preferred for preventing data loss if streaming processing encounters any problem (network connection, server inaccessibility, etc.). Kafka provides semantic (exactly-once) to prevent data duplication as well.
In this architecture, the sole thing which can create a risky situation in term of offset management is checkpoint management of Spark Streaming API. Kafka consumer API, inherently, stores the processed offsets in a special topic on Kafka cluster. Similarly, Spark’s checkpoint mechanism simulates this functionality itself. But, according to its documentation, there are some drawbacks and it does not give a guarantee as a perfect solution.
This use case has been tested on Cloudera Quickstart 5.13. Dependency versions of the project may vary different Kafka, Spark, and HBase versions. Below versions has been used on Maven POM file:
<dependency>
<groupId>org.apache.spark</groupId>
<artifactId>spark-streaming_2.11</artifactId>
<version>2.4.0</version>
</dependency>
<dependency>
<groupId>org.apache.kafka</groupId>
<artifactId>kafka_2.11</artifactId>
<version>2.4.1</version>
</dependency>
<dependency>
<groupId>org.apache.hbase</groupId>
<artifactId>hbase-client</artifactId>
<version>2.2.4</version>
</dependency>
Three IoT devices are simulated. Each device will generate data according to the following template
{
"data":{
"deviceId":"11c1310e-c0c2-461b-a4eb-f6bf8da2d23c",
"temperature":12,
"location":{
"latitude":"52.14691120000001",
"longitude":"11.658838699999933"
},
"time":"1509793231"
}
}
The properties are defined in the following way
Property Name | Data Type | Comment |
devideId | UUID | The unique ID of the device sending the data |
temperature | Integer | The temperature measured by the device |
latitude | Long | The latitude of the position of the device |
longitude | Long | the longitude of the position of the device |
time | Timestamp | The time of the signal as a Unix timestamp. |
It is assumed that the simulator should simulate three different devices and needs to send a signal to Apache Kafka every second.
In order to store streaming data on HBase, “iot” named table has been created using hbase shell.
Create ‘iot’,’rawdata’,’data’
Let’s check the new table on Hbase shell
describe 'iot'

Streaming application has been written using Java. The data generator (DataGenerator) class implements the Runnable interface for multithreading behavior.
package com.company.iot.data.producer; import com.company.iot.data.model.json.Data; import com.company.iot.data.util.JsonGenerator; import com.company.iot.data.util.ProducerConfigCreator; import com.google.gson.Gson; import org.apache.kafka.clients.producer.KafkaProducer; import org.apache.kafka.clients.producer.ProducerRecord; import org.slf4j.Logger; import org.slf4j.LoggerFactory; /** * DataGenerator class provides dummy iot raw data for each second * @author Onur Tokat */ public class DataGenerator implements Runnable { private static final Logger LOG = LoggerFactory.getLogger("DataGenerator"); private Gson gson = new Gson(); private String topicName; public DataGenerator(String topicName) { this.topicName = topicName; } @Override public void run() { //private ProducerRecord<String, String> producerRecord = null; KafkaProducer<String, String> kafkaProducer = new KafkaProducer<>(ProducerConfigCreator.getConfig()); //Infinite loop for producing data while (true) { Data data = gson.fromJson(JsonGenerator.generate(), Data.class); kafkaProducer.send(new ProducerRecord<>(topicName, data.getDeviceId(), JsonGenerator.generate())); kafkaProducer.flush(); try { //on each second, data will be produced Thread.sleep(1000); } catch (InterruptedException e) { LOG.info("Error occurred when producing data", e); } } } }
Each IoT data are gathered into one Kafka topic (device1). Three separate Kafka topics can be used, it is up to developer way.
package com.company.iot.data.streaming; import com.company.iot.data.model.json.Data; import com.google.gson.Gson; import org.apache.hadoop.conf.Configuration; import org.apache.hadoop.hbase.HBaseConfiguration; import org.apache.hadoop.hbase.TableName; import org.apache.hadoop.hbase.client.Put; import org.apache.hadoop.hbase.spark.JavaHBaseContext; import org.apache.hadoop.hbase.util.Bytes; import org.apache.kafka.clients.consumer.ConsumerRecord; import org.apache.kafka.common.serialization.StringDeserializer; import org.apache.spark.SparkConf; import org.apache.spark.api.java.JavaSparkContext; import org.apache.spark.api.java.function.Function; import org.apache.spark.streaming.Duration; import org.apache.spark.streaming.api.java.*; import org.apache.spark.streaming.kafka010.ConsumerStrategies; import org.apache.spark.streaming.kafka010.KafkaUtils; import org.apache.spark.streaming.kafka010.LocationStrategies; import org.apache.hadoop.fs.Path; import org.slf4j.Logger; import org.slf4j.LoggerFactory; import java.time.Instant; import java.time.LocalDateTime; import java.time.format.DateTimeFormatter; import java.util.*; /** * JavaHBaseStreaming class provides spark streaming application * from Kafka topic to HBase table. * * @author Onur Tokat */ public class JavaHBaseStreaming { private static final Logger LOGGER = LoggerFactory.getLogger("JavaHBaseStreaming"); private static Gson gson = new Gson(); private static final String TABLE_NAME = "iot"; private static DateTimeFormatter formatter = DateTimeFormatter.ofPattern("yyyy-MM-dd'T'HH:mm:ss.SSS"); private JavaHBaseStreaming() { } public static void main(String[] args) { SparkConf sparkConf = new SparkConf().setMaster("local[*]").setAppName(UUID.randomUUID().toString()); JavaSparkContext jsc = new JavaSparkContext(sparkConf); //Kafka configuration Map<String, Object> kafkaParams = new HashMap<>(); kafkaParams.put("bootstrap.servers", "localhost:9092"); kafkaParams.put("key.deserializer", StringDeserializer.class); kafkaParams.put("value.deserializer", StringDeserializer.class); kafkaParams.put("group.id", "use_a_separate_group_id_for_each_stream"); kafkaParams.put("auto.offset.reset", "latest"); kafkaParams.put("enable.auto.commit", false); Collection<String> topics = Arrays.asList("device1"); try { JavaStreamingContext jssc = new JavaStreamingContext(jsc, new Duration(1000)); JavaInputDStream<ConsumerRecord<String, String>> stream = KafkaUtils.createDirectStream( jssc, LocationStrategies.PreferConsistent(), ConsumerStrategies.Subscribe(topics, kafkaParams) ); JavaDStream<String> javaDstream = stream.map(ConsumerRecord::value); //HBase configuration Configuration conf = HBaseConfiguration.create(); conf.addResource(new Path("/etc/hadoop/conf.cloudera.hdfs/core-site.xml")); conf.addResource(new Path("/etc/hbase/conf.cloudera.hbase/hbase-site.xml")); JavaHBaseContext hbaseContext = new JavaHBaseContext(jsc, conf); hbaseContext.streamBulkPut(javaDstream, TableName.valueOf(TABLE_NAME), new PutFunction()); jssc.start(); try { jssc.awaitTermination(); } catch (InterruptedException e) { LOGGER.error("Error occurred when java spark streaming context operation: ", e); } } finally { jsc.stop(); } } public static class PutFunction implements Function<String, Put> { private static final long serialVersionUID = 1L; public Put call(String v) { Data data = gson.fromJson(v, Data.class); //composite rowkey with deviceId and time Put put = new Put(Bytes.toBytes(data.getDeviceId() + "_" + data.getTime())); put.addColumn(Bytes.toBytes("rawdata"), Bytes.toBytes("rawValue"), Bytes.toBytes(v)); put.addColumn(Bytes.toBytes("data"), Bytes.toBytes("deviceId"), Bytes.toBytes(data.getDeviceId())); put.addColumn(Bytes.toBytes("data"), Bytes.toBytes("temperature"), Bytes.toBytes(String.valueOf(data.getTemperature()))); put.addColumn(Bytes.toBytes("data"), Bytes.toBytes("latitude"), Bytes.toBytes(String.valueOf(data.getLocation().getLatitude()))); put.addColumn(Bytes.toBytes("data"), Bytes.toBytes("longitude"), Bytes.toBytes(String.valueOf(data.getLocation().getLongitude()))); put.addColumn(Bytes.toBytes("data"), Bytes.toBytes("time"), Bytes.toBytes(getFormattedDateTime(data.getTime()))); return put; } } /** * getFormattedDateTime method * * @param value long value * @return String of formatted local date time */ private static String getFormattedDateTime(Long value) { Instant instant = Instant.ofEpochSecond(value); return (LocalDateTime.ofInstant(instant, TimeZone.getDefault().toZoneId()).format(formatter)); } }
In order to specify Hadoop configurations, paths of the core-site.xml and HBase-site.xml files have been added to the Hadoop configuration object.
Each second, the polled record of the Kafka topic is added to the Hbase column as byte array invoking call method of the PutFunction class.
Another way for sinking data is to use SparkSession. For using SparkSession effectively, It makes sense to select the Scala language.
package com.company.iot.data.spark import java.util.UUID import org.apache.log4j.{Level, Logger} import org.apache.spark.sql.SparkSession import org.apache.spark.sql.streaming.{OutputMode, Trigger} import org.apache.spark.sql.types.{IntegerType, LongType, StringType, StructField, StructType} /** * @author Onur Tokat * ScalaStreamingApp provides streaming application * using SparkSession object for using SparkSQL API. * Hortonworks shc API is used as HBase connector. * //FIXME current API have bug which is java.lang.NoSuchMethodError: org.json4s.jackson.JsonMethods$.parse */ object ScalaStreamingApp { def main(args: Array[String]): Unit = { Logger.getLogger("org").setLevel(Level.ERROR) if (args.length != 1) { println("Topic name should be entered as argument") System.exit(-1) } val spark = SparkSession.builder().appName(UUID.randomUUID().toString) .config("spark.sql.streaming.unsupportedOperationCheck", false) .getOrCreate() // creating stream DataFrame from Kafka Topic val df = spark .readStream .format("kafka") .option("kafka.bootstrap.servers", "localhost:9092") .option("subscribe", args(0)) .option("zookeeper.connect", "localhost:2181") .option("startingOffsets", "earliest") .option("max.poll.records", 10) .option("failOnDataLoss", false) .load() df.printSchema() //creating schema for iot data val dataSchema = StructType(List( StructField("deviceId", StringType, true), StructField("temperature", IntegerType), StructField("location", StructType(List( StructField("latitude", LongType), StructField("longitude", LongType)))), StructField("time", LongType) )) //creating schema for HBase data def catalog = s"""{ |"table":{"namespace":"default", "name":"iot"}, |"rowkey":"deviceId", |"columns":{ |"deviceId":{"cf":"rowkey", "col":"deviceId", "type":"string"}, |"value":{"cf":"rawdata", "col":"rawValue", "type":"string"}, |"deviceId":{"cf":"data", "col":"deviceId", "type":"string"}, |"deviceId":{"cf":"data", "col":"temperature", "type":"integer"}, |"latitude":{"cf":"data", "col":"latitude", "type":"long"}, |"longitude":{"cf":"data", "col":"longitude", "type":"long"}, |"time":{"cf":"data", "col":"time", "type":"long"} |} |}""".stripMargin import org.apache.spark.sql.functions._ //transformation on raw data val formattedDF = df.withColumn("formatted", from_json(col("value").cast(StringType), dataSchema)) .withColumn("rawValue", col("value").cast(StringType)) .select("rawValue", "formatted.deviceId", "formatted.temperature", "formatted.location.latitude", "formatted.location.longitude", "formatted.time") .withColumn("time", from_unixtime(col("time").cast(LongType), "yyyy-MM-dd'T'HH:mm:ssXXX")) formattedDF.printSchema() //write data to HBase val streaming = formattedDF.writeStream .queryName("hbase writer") .format("com.company.iot.data.spark.HBaseSinkProvider") .option("checkpointLocation", "/user/cloudera/checkpoint_onur") .option("hbasecat", catalog) .outputMode(OutputMode.Update()) .trigger(Trigger.ProcessingTime("30 seconds")) .start streaming.awaitTermination() spark.stop() } }
HBase Sink class:
package com.company.iot.data.spark import org.apache.hadoop.hbase.spark.datasources.HBaseTableCatalog import org.apache.spark.internal.Logging import org.apache.spark.sql.execution.streaming.Sink import org.apache.spark.sql.{DataFrame} class HBaseSink(options: Map[String, String]) extends Sink with Logging { // String with HBaseTableCatalog.tableCatalog private val hBaseCatalog = options.get("hbasecat").map(_.toString).getOrElse("") override def addBatch(batchId: Long, data: DataFrame): Unit = synchronized { val df = data.sparkSession.createDataFrame(data.rdd, data.schema) df.write .options(Map(HBaseTableCatalog.tableCatalog->hBaseCatalog, HBaseTableCatalog.newTable -> "5")) .format("org.apache.spark.sql.execution.datasources.hbase").save() } }
HBase sink provider:
package com.company.iot.data.spark import org.apache.spark.sql.SQLContext import org.apache.spark.sql.execution.streaming.Sink import org.apache.spark.sql.sources.{DataSourceRegister, StreamSinkProvider} import org.apache.spark.sql.streaming.OutputMode class HBaseSinkProvider extends StreamSinkProvider with DataSourceRegister { def createSink( sqlContext: SQLContext, parameters: Map[String, String], partitionColumns: Seq[String], outputMode: OutputMode): Sink = { new HBaseSink(parameters) } def shortName(): String = "hbase" }
In fact, Spark SQL API have not got hbase datasource connector class.
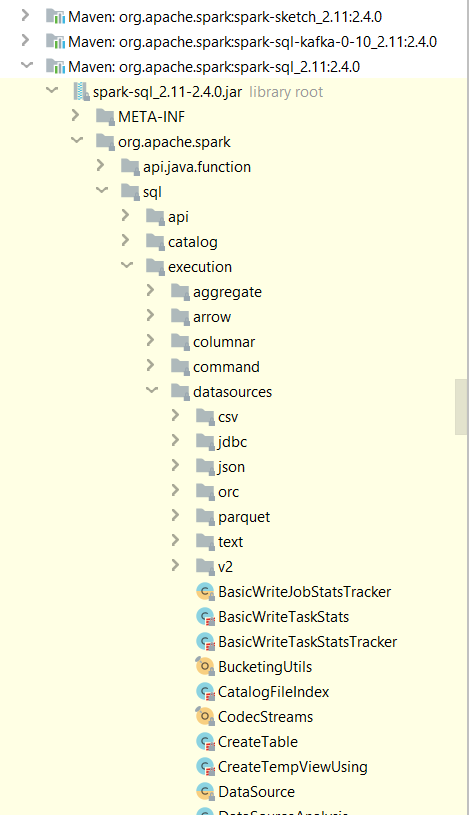
But, Hortonworks’ developers developed a datasource for connecting Hbase.
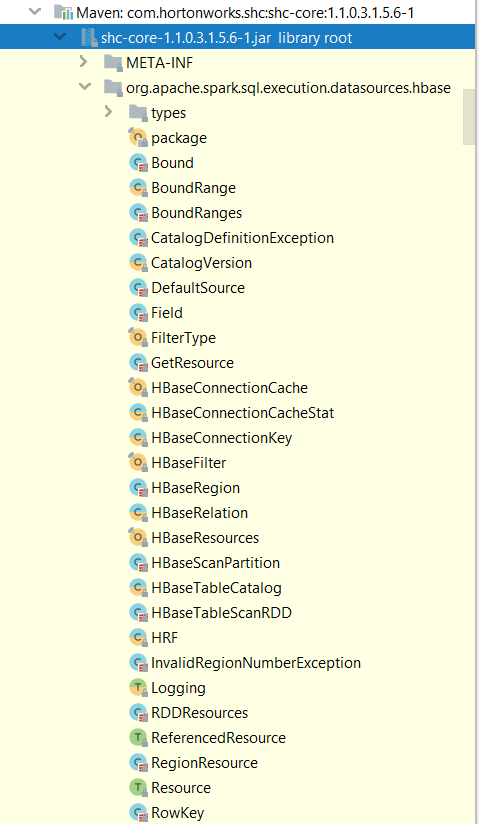
In the HBaseSink class, the datasource path is used, as if Spark SQL API have a hbase datasource connector.
val df = data.sparkSession.createDataFrame(data.rdd, data.schema) df.write .options(Map(HBaseTableCatalog.tableCatalog->hBaseCatalog, HBaseTableCatalog.newTable -> "5")) .format("org.apache.spark.sql.execution.datasources.hbase").save()
Project code can be downloaded from my Github